Data Science or Data Analytics: Similarities and Differences
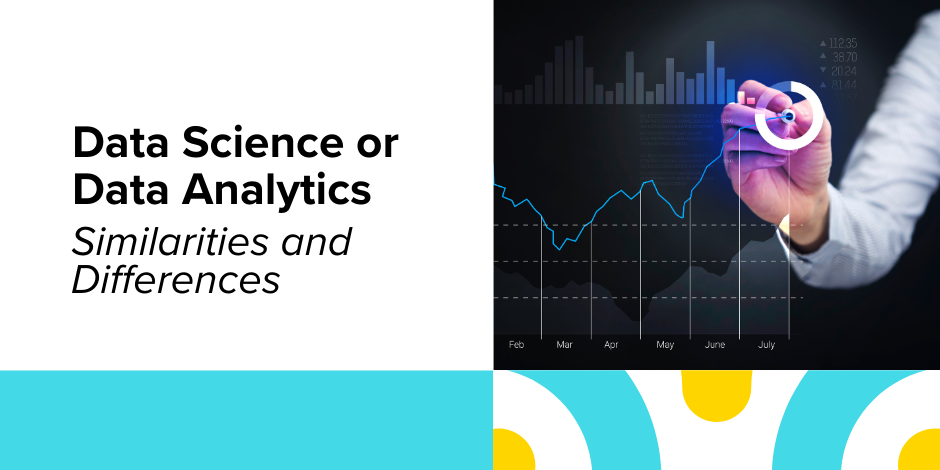
Stay Informed With Our Weekly Newsletter
Receive crucial updates on the ever-evolving landscape of technology and innovation.
As the digital landscape advances, the significance of both data science and data analytics is becoming more evident. Projections indicate that the big data analytics market will soar to $349.56 billion by 2024.
Businesses, governments, and organisations worldwide are harnessing the power of data to drive decision-making, improve operations, and gain a competitive edge.
Two key disciplines that have emerged in this data-driven landscape are data science or data analytics.
While the terms data science or data analytics are often used interchangeably, they have distinct characteristics and roles within the broader field of data management.
Understanding data science and data analytics
Data science is a multidisciplinary field that uses scientific methods, processes, algorithms, and systems to extract knowledge and insights from structured and unstructured data.
It involves a wide range of techniques and theories drawn from many fields within the context of mathematics, statistics, computer science, and information science.
On the other hand, Data Analytics is the process of examining, cleaning, transforming, and modelling data to discover useful information, draw conclusions, and support decision-making.
It is a more focused version of data science with an emphasis on the interpretation of historical data to uncover potential trends and gauge the effectiveness of certain decisions or events.
Similarities between data science and data analytics
Role in decision making
Either data science or data analytics plays a crucial role in decision-making. They provide valuable insights that can guide strategy, improve efficiency, and drive innovation.
By leveraging these insights, organisations can make more informed decisions, predict future trends, and better understand their customers and competitors.
Moreover, data science or data analytics involves the use of statistical methods and data visualisation techniques to interpret and present data in a meaningful way.
This enables stakeholders to understand complex data sets and make data-driven decisions.
Use of tools and techniques
Data science and data analytics also share similarities in the tools and techniques they use. Both fields utilise programming languages like Python and R for data manipulation and analysis.
They also use SQL for data extraction and manipulation, and tools like Tableau for data visualisation.
Furthermore, both fields involve the use of machine learning techniques for predictive analysis.
Machine learning, a subset of artificial intelligence, enables systems to learn and improve from experience without being explicitly programmed.
Differences between data science and data analytics
Scope and depth
While both data science and data analytics deal with data, the scope and depth of their work differ significantly. Data science is a more holistic field that encompasses data analytics.
It involves extracting, preprocessing, analysing, and visualising data. It also includes predictive modelling and machine learning.
In contrast, data analytics is more focused and less comprehensive. It primarily involves the analysis of historical data to identify trends and patterns.
While data analytics may utilise some aspects of machine learning, it does not delve as deeply into predictive modelling and algorithm development as Data Science does.
Objective and approach
The objective and approach of data science and data analytics also differ. Data Science is often exploratory and hypothesis-driven.
It seeks to ask questions and find potential pathways of analysis.
It’s about uncovering hidden insights and finding potential trends and relationships that can lead to actionable insights.
On the other hand, data analytics is more about answering specific questions and solving specific problems. It’s about providing concrete and actionable insights based on historical data.
It’s less about exploration and more about finding direct, actionable answers.
Choosing between data science or data analytics
Choosing between data science or data analytics largely depends on your specific needs and objectives.
If you’re looking for a comprehensive, exploratory approach that can uncover hidden insights and predict future trends, Data Science may be the better choice.
It’s ideal for organisations that have large amounts of unstructured data and want to leverage this data to drive innovation and strategic decision-making.
However, if you’re looking for a more focused, problem-solving approach that can provide actionable insights based on historical data, Data Analytics may be more suitable.
It’s ideal for organisations that want to understand their past performance, identify trends, and make data-driven decisions.
Conclusion
When choosing data science or data analytics it’s important to consider that although they share some similarities, they have very distinct roles and characteristics.
Understanding these differences can help organisations choose the right approach for their data management needs and leverage the power of data to drive success.
Want to learn more about data science or data analytics? Download a copy of the Institute of Data’s comprehensive Data Science & AI Course Outline for free.
Alternatively, we invite you to schedule a complimentary career consultation with a member of our team to discuss the program in more detail.